Comprehensive Guide to Retriever-Augmented Generation (RAG): Part 1 – Why Needed?
Table of Contents
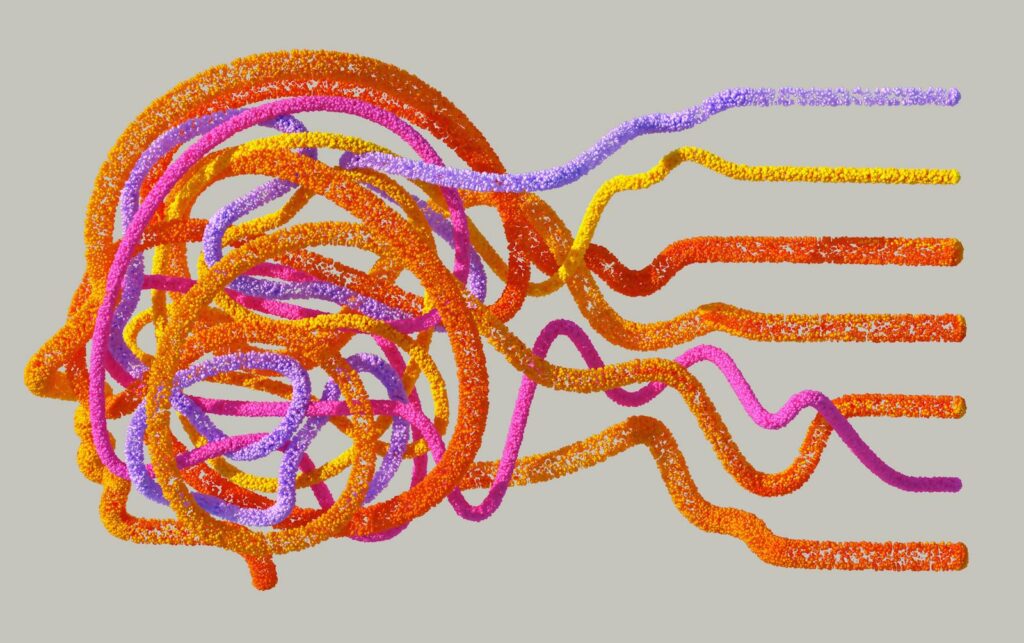
In the rapidly evolving field of natural language processing (NLP), one innovation stands out for its ability to enhance the generation of human-like text: Retriever-Augmented Generation, aka. RAG. This groundbreaking technology leverages the power of retrieval-based mechanisms alongside advanced language models to produce responses that are not only relevant but also rich in information and contextually appropriate. The inception of RAG marks a significant milestone, bridging the gap between voluminous data retrieval and the nuanced creation of text, thus paving the way for a new era in AI-driven communication.
Retriever-Augmented Generation (RAG) addresses several limitations present in traditional natural language processing (NLP) models, making it a necessary evolution in the quest for more accurate, relevant, and contextually rich text generation
Enhanced Accuracy and Relevance
Traditional NLP models, especially those based on pre-trained language models like GPT or BERT, generate responses based on the knowledge they were trained on, which may be outdated or limited in scope. RAG, by incorporating a retriever component that sources information from a vast, up-to-date knowledge base, ensures that the generated responses are not only relevant but also accurate and informed by the latest data.
Contextual Understanding
RAG models excel at understanding and incorporating context into their responses. By retrieving information that is specifically relevant to the query at hand, these models can produce answers that are deeply aligned with the user’s intent and the nuances of the question, leading to more satisfactory and precise interactions.
Scalability and Flexibility
As knowledge evolves, so too does the information accessible to RAG models through their retriever component. This means that RAG systems can easily adapt to new information, trends, and data without the need for extensive retraining. This scalability and flexibility make RAG a valuable tool for applications that require up-to-date information, such as news aggregation, research assistance, and customer service.
Depth of Knowledge
While traditional models are limited by the data they were trained on, RAG can draw upon a practically unlimited pool of information. This depth of knowledge allows RAG to answer a broader range of questions with a level of detail and specificity that pre-trained models simply cannot match.
Efficiency and Cost-Effectiveness
Updating traditional NLP models to keep pace with new information can be both time-consuming and costly, requiring retraining or fine-tuning with large datasets. RAG’s ability to dynamically retrieve information reduces the need for frequent updates, making it a more efficient and cost-effective solution for applications that require access to the latest information.
Enhanced Creativity and Coverage
RAG models can generate more creative and varied responses by leveraging diverse sources of information. This enhances the user experience, providing answers that are not only informative but also engaging and comprehensive.
* * * * * * * * * *
If Generative AI technologies pique your interest, the following article might provide you with some insights.
Leave a Reply